The promise of AI is plain and simple: greater product development efficiency and faster time to market. Products are becoming more complex, yet engineering teams don’t have more time. Using historical or current engineering test data to train self-learning models, today’s visionary engineering leaders are reducing testing time and simulations for products with even the most intractable physics, increasing competitiveness and speeding time to market.
The release of ChatGPT has been a quantum shift in the public’s perception of what artificial intelligence can do and has sparked a boom in interest. GPT4 can now match human intelligence simply by having trained a neural network of enormous size on an enormous amount of data.
The story that deep learning can outperform other methods and even surpass human intelligence is not new. By training reinforcement learning methods, Google beat the world champion at Go. Later, they used deep learning to understand protein folding, an immensely complicated scientific problem. This last accomplishment is crucial to knowing where AI should be applied in engineering: to solve intractable physics problems.
Most research labs at top universities and leading companies are currently exploring how to solve materials, turbulence, chemical, or other physically intractable problems with AI.
The biggest challenge they must overcome is collecting and using test data. In a recent Forrester survey of engineering leaders, 50% said they do not analyze historical test data, and 54% found recording and storing test data properly for others to use later extremely or very challenging. Looking ahead, the opportunity is to rethink how you are working and understand where and how AI complements or replaces your current tools and workflows.
The same Forrester survey also found that 71% of engineering leaders need to find ways to speed product development to stay competitive, and the majority (67%) also feel pressure to adopt AI. And yet, in the areas where AI technology is being applied, R&D teams are realising incredible innovations in new product development. The study found that those who have adopted AI are more likely to achieve increased revenue, profitability, and competitiveness for their employers.
Let’s look at a few examples.
Improving vehicle acoustics with AI
Kautex-Textron is a top 100 automotive supplier to global OEMs. The Kautex-Textron validation engineering team used AI to enhance vehicle acoustics by solving one of their most complex engineering challenges with fuel ‘sloshing’ while reducing design iterations, prototyping, and testing costs.
The core of the Kautex engineers’ challenge was to reliably understand the relationship between the properties of the fuel tank, the test parameters, and the resulting sloshing noise – an intractable physics procedure typically requiring multiple physical tests with prototype tank shapes filled at differing levels.
Dr. Bernhardt Lüddecke, Global Director of Validation, Kautex-Textron, said: “With the Monolith machine learning method, we solved the challenge and reduced design iteration times and prototyping and testing costs. The software reduces design analysis time from days to minutes with improved accuracy. We are thrilled with the results and confident we have found a way to improve future design solutions.”
Leveraging the power of AI to accurately predict sloshing noise generated when a vehicle decelerates, the ground-breaking work opened a world of opportunities for Kautex-Textron engineers to expand the application of AI to solve further engineering challenges in the era of electrification.
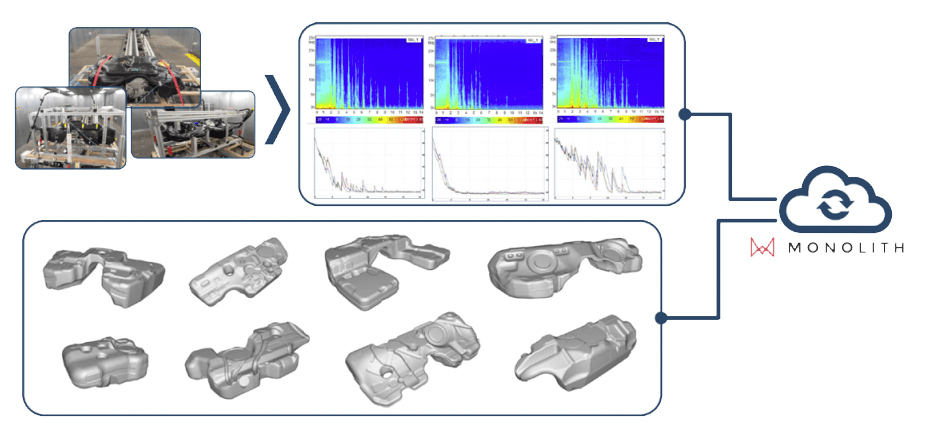
Honeywell reduces product development time by 25%
The product innovation team at Honeywell is always exploring new methods to design products faster while meeting exceedingly high-quality standards and creating products that customers can trust in times of high energy bills and fluctuating prices. Often, these products, such as smart meters to measure energy consumption, involve highly complex physics, such as gas fluid dynamics, that require extensive simulation and physical testing to understand.
The engineering team at Honeywell collaborated with our engineers to explore how advanced machine learning methods could be used to understand and predict complex product behaviors faster and in extreme operation conditions. Within days of using no-code AI software tailored explicitly to engineering applications, they found new insights that delivered real business value.
Before using AI, Honeywell followed a traditional and familiar development process that involved physically and virtually testing of many different product variables to arrive at a product design as fast as possible that was suitable for manufacture. This process typically takes 18 months to ensure that the calibration error is below the minimum legally required 1%. With AI, they could achieve this 25% faster.
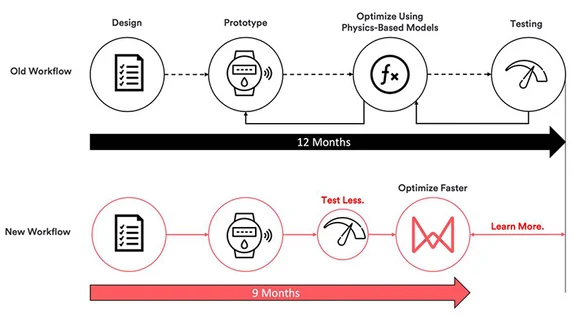
Jota Sports cuts car setup time by 50%
Race cars are complicated. The biggest area of complexity is the massive amount of data engineers must deal with. For instance, during race weekends, Jota Sports, a British sports car racing team with a history of punching above its weight, produces 7500 data points per second. That’s 27 million data points per hour, and races last much more than one hour – Le Mans notably lasts 24.
Le Mans is arguably one of the most elite car races in the world. For a small racing team like Jota, with a limited budget and time to create a car that can compete on this stage, their visionary engineers had to think differently. Using traditional methods to model the car and validate the prototype under every conceivable condition was not only cost-prohibitive but humanly impossible.
By using AI, Jota engineers can better understand and predict the aerodynamics of their cars by building self-learning models. As a result, they have reduced the number of simulations and tests by 50%, cut car time-to-setup in half, and achieved a 66% reduction in overall costs.
Solving highly complex physics problems
These examples make clear that self-learning models are becoming a standard tool for engineering. As AI becomes a trusted part of the product development process, we expect engineers across all industries to reduce by 30-60% the verification and validation tests that today take weeks or months. Of course, there are areas in which AI is more suited than others; we believe its greatest promise is firmly located in deeply complex engineering problems where the physics is intractable, and the number of parameters is extensive.
As the non-linear, high-dimensional complexity of products in these industries becomes more and more challenging to understand, engineers find themselves in a dilemma: either conducting excessive tests to cover all possible operating conditions or running insufficient tests that risk the omission of critical performance parameters.
AI is already reducing validation tests by up to 60%
As data science research continues, new AI capabilities empower engineers to realize even more value. For instance, using robust active learning technology, engineers can now build models inside Monolith that will automatically provide a recommended list, in ranked order, of the critical tests to run and which to skip.
This capability, called the ‘next test recommender,’ works for any complex system engineers trying to safely explore the design space, such as battery or fuel cell cooling system calibration. In one fuel cell use case, an engineer trying to configure a fan to provide optimal cooling for all driving conditions had a test plan for this highly complex, intractable application that included running a series of 129 tests. When this test plan was inserted into the software, it returned a ranked list of what tests should be carried out first. Out of 129 tests, as shown in Fig 3., the platform recommended the last test – number 129 – should be among the first five to run and that 60 tests are sufficient to characterize the full performance of the fan, a 53% reduction in testing.

While available, open-source AI methods don’t allow an engineer to influence the test plan, a critically unique aspect of the ‘next test recommender’ technology is that it will enable human-in-the-loop inspection of the selected experiments, granting a domain expert user oversight of the system, combining their expertise and domain knowledge with the power of machine learning without any knowledge of AI or coding.
AI won’t replace you; engineers using AI will
Machine learning is becoming an increasingly important part of our personal and business lives, either as a conscious decision by the user or subtly through the basic tools we use daily. There’s understandable anxiety among knowledge workers around AI, but we see much more upside than potential risk of downside. This technology will foster greater engineering creativity, create many more new jobs, and improve how engineers develop great products.
And we believe by 2030, every engineer will be an AI engineer. These AI engineers will not need to be Python coders or data scientists, just domain experts in their field. On our way to this aspirational goal, we’ve defined a near-term vision: by 2026, we will empower 100,000 visionary engineers to use machine learning to cut their product development cycle in half and help their employers be more profitable.
Are you one of the hundred thousand?
Leave a Reply
You must be logged in to post a comment.